Revolutionizing Position Estimation with CNN and Radio Signal Analysis
Discover how our CNN-based approach to radio signal analysis outperforms traditional methods in complex multipath scenarios, showcasing machine learning's potential to revolutionize high-precision positioning.
At the Mobile World Congress Barcelona 2018, our demonstrator showcased a groundbreaking machine learning model, a deep convolutional neural network (CNN), for high-precision positioning in Industrial 5G IoT contexts. Unlike conventional signal processing, our ML-powered approach consistently tracked objects amidst complex multipath distortions, a significant advancement presented by the team at Fraunhofer IIS.
Understanding Channel Impulse Response
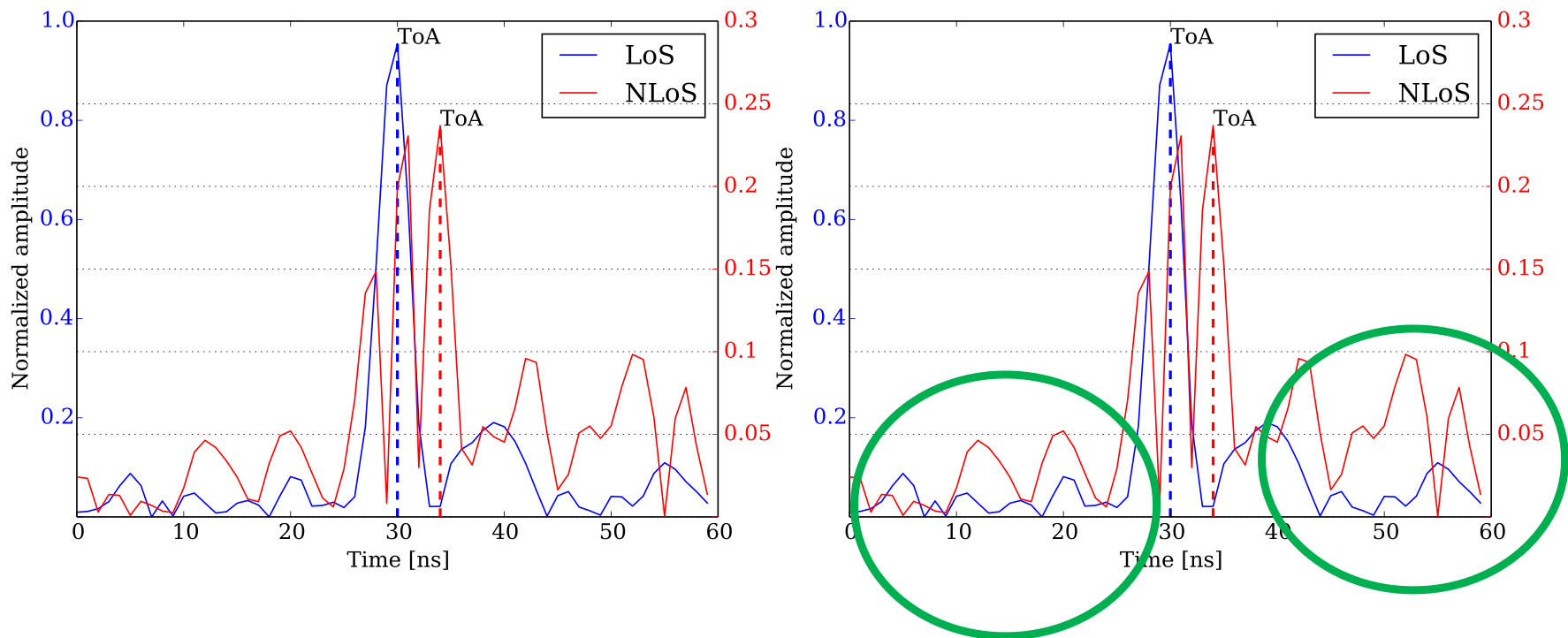
Channel impulse response analysis reveals the challenges of traditional time-of-arrival (TOA) estimation in multipath scenarios. Our CNN model, however, leverages the full spectrum of signal information, including multipath reflections, to achieve unprecedented positioning accuracy.
Advanced Data Processing Techniques
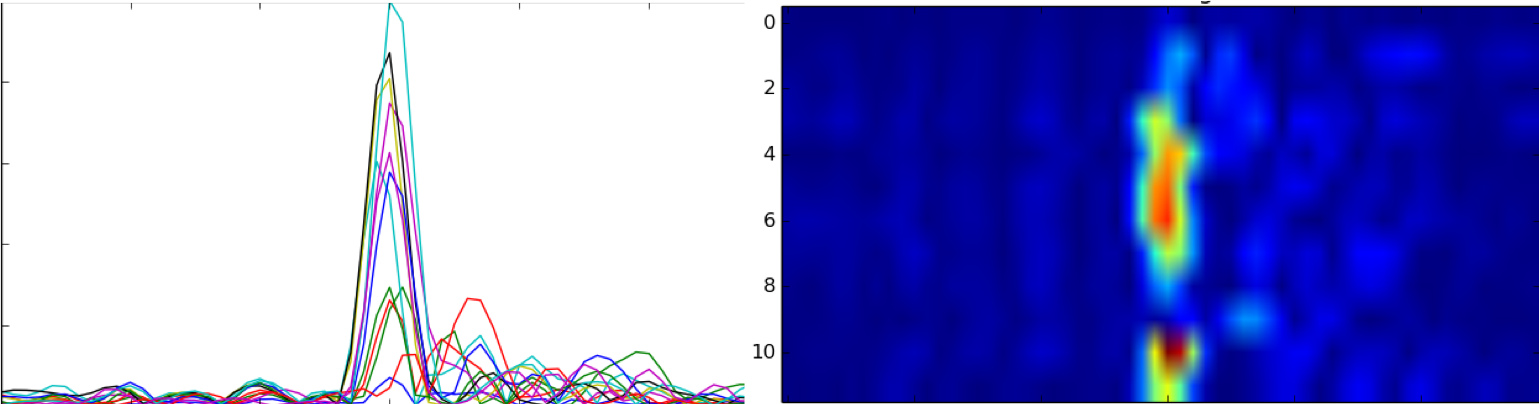
By transforming real and imaginary signal components from multiple antennas into a 2D image format, our CNN model interprets complex signal patterns, enhancing the accuracy of position estimation across various antenna configurations.
Innovative Datasets and Training Methodologies
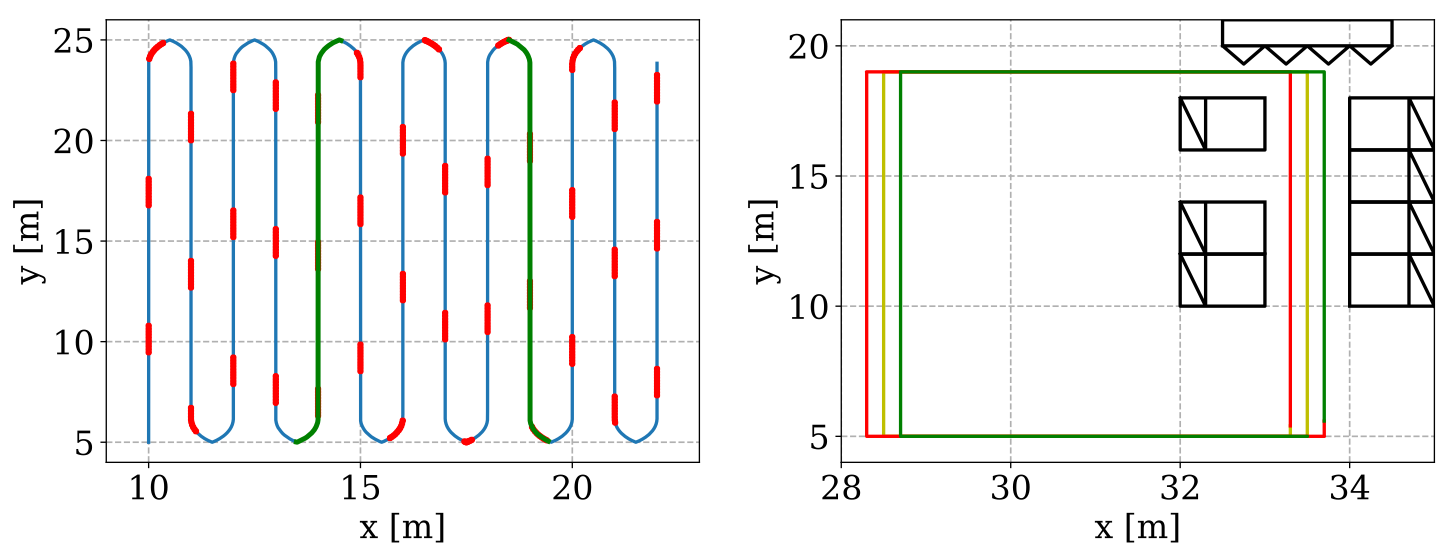
Our unique dataset includes meticulously designed trajectories to test the CNN model against rigorous multipath conditions, demonstrating its capability to interpolate and extrapolate positioning data with high precision.
Groundbreaking Results
Our CNN model substantially outperforms traditional signal processing in multipath scenarios, as shown in our comprehensive evaluation:
- Short Slices: Achieved a mean absolute error (MAE) of only 0.265m.
- Long Slices: Demonstrated robustness with a MAE of 0.339m.
- Multipath vs. Line of Sight: In multipath conditions, the CNN model significantly reduced error margins compared to Extended Kalman Filter (EKF) based methods.
Concluding Thoughts
This project not only garnered academic accolades but also marked a significant leap forward in radio wave-based positioning technologies. It underscores the transformative potential of convolutional neural networks in overcoming the limitations of traditional signal processing techniques.